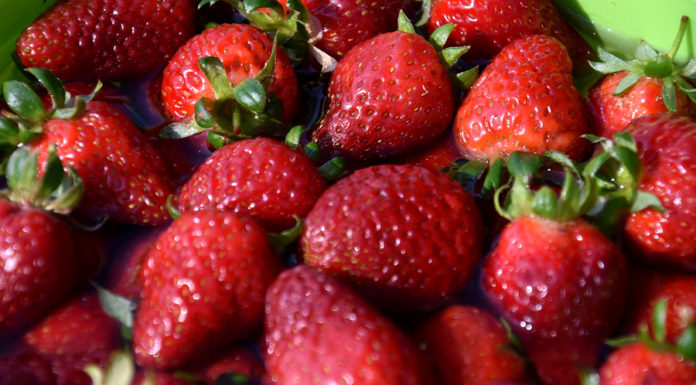
The problem of food waste has become front and center for consumers and companies alike. Just last week, a study in PLOS ONE revealed that U.S. consumers waste an astonishing 1 pound of food per person per day. In total, that represents:
- 30% of daily calories available for consumption,
- 25% of daily food (by weight) available for consumption, and
- 7% of annual cropland acreage.
And that’s just what gets thrown out of consumers’ fridges. Waste occurs throughout the supply chain, from the farm to the processor to the retailer.
Recently, we’ve seen several new technologies arise to solve this problem.
Earlier this year, we interviewed Christine Moseley of Full Harvest, an online marketplace that allows food and beverage processors to purchase imperfect or surplus produce directly from farms. For this article, we looked further down the supply chain at a new technology that can help food companies reduce waste by improving their food inspection system.
AgShift is designing world’s most advanced, autonomous food inspection system that uses Deep Learning — a subset of Artificial Intelligence (AI) — to automate the food inspection system. It’s an innovative mobile-based software solution that organizations can use to standardize inspections by “providing objective, consistent, and standardized quality assessment across the organization — every single time.” The goal is not only to reduce food waste, but also to save companies money in recovery costs and help them maintain their reputation for quality products.
To learn more about AgShift and the technology behind it, Food Industry Executive spoke with the company’s founder and CEO, Miku Jha.
Let’s start with your background. What’s the 30,000-foot view of your career and your interest in food and ag tech?
MJ: I grew up on a mango farm in India. My family belonged to a smallholder farmers community.
As far as passion goes, I’m a technologist. I like to build innovative products, and I’ve done that multiple times over, with companies from startups to IBM. AgShift is the fourth company I’ve started, but the first one in food and ag.
Back in 2016, I was leading the Internet of Things initiative at IBM and thinking about my next venture. If you remember, that was a year California was going through a tough time in terms of drought. I started to think about the challenges facing these communities. That’s when I decided to do something in food and ag, so I could apply what I know about technology and give it back to this community.
With that notion, I relocated from Manhattan back to Silicon Valley and started AgShift in Q2 of 2016. So much innovation is happening in technology all across the world, but there are very few eyes and minds focused on applying that innovation to food and ag. I believe that we should attempt to close that gap.
How did you come up with the idea of automating food quality grading?
As you look at food and ag, there are many complex, noble challenges. You read a lot about how we have to double or triple the production to feed the growing population. But there’s also the other side of it, reducing food wastage. Either you make more or you waste less.
We started researching what causes waste, specifically, what percentage of wastage is contributed on the supply chain side and why. There, we saw that many times, inconsistent inspections or quality assessments lead to the rejection of food. The inconsistencies usually happen because of the subjective nature of how the inspections or quality assessments are done.
Anything that’s subjective, you can’t make 100% digital, but you can augment by applying digitization and data to make it a data-driven assessment, as opposed to just a biased call. That’s how we decided to focus on how we can improve how quality assessment is done across the supply chain, all the way from farm to fork.
We focus on empowering food quality inspectors in the food industry with the ability to provide fast and consistent food quality assessment that for decades has been relegated to inaccurate, ad-hoc processes with cumbersome paperwork. Our IT innovations target the $22B food inspection market by radically changing the time and skill set needed to empower field workers to accurately perform food quality assessment and grading across multiple points in the supply chain.
How did you get started?
I started by visiting farms. I drove 90 miles in all directions from Silicon Valley to see what really goes on. Yes, I had some exposure growing up in India, but it’s different here.
I have a very strong background when it comes to building enterprise software, building technology. Switching into food, I absolutely started from scratch. I didn’t know people in the ecosystem. So, I did my own crash course for first six to eight months by driving to random farms. Slowly, I started realizing where all the pain points are and where we can start addressing those challenges.
How does AgShift address those challenges?
AgShift uses applies artificial intelligence, more specifically deep learning, to the food inspection process.
The best analogy is self-driving cars. After the car hits a pothole 1000 times, it realizes it has to do something different. We’re applying that same cutting-edge technology to self-grading strawberries, or self-grading tomatoes, or self-grading nuts.
Deep learning works by training models on different attributes or parameters. Let’s say I collect a large number of images of strawberries that are bruised or have decay or mold. I feed those into the model. After I feed it enough images, it starts to understand, “this is decay,” “this is mold.”
That’s what’s happening under the hood. The result is that the whole assessment is done by a massive network. You take a picture of a sample, and the software can tell you, for example, the percentage of bruising, the average color, the average size, the percentage of decay, and so on. The system has no mood swings and no biases. It’s objective, which means it’s 100% consistent.
What types of products can AgShift inspect?
From a technology perspective, it can do anything that has a visual aspect to it. If your eyes can see it, then a camera can capture it, and if a camera can capture it, then my software can learn it.
For now, we’re looking at three main categories of food. First is fresh produce — this is where we started. The second category is edible nuts, and the third is seafood, because seafood has a very high rate of perishability and being rejected.
How long does it take to train up the model on a new type of food?
It depends on the commodity. We’re doing a lot of innovation in this space right now.
For some commodities, like strawberries, the models are ready to use. We started with strawberries because it’s one of the more difficult commodities to solve for — everything is red. We’re aggressively working on other commodities.
Usually, the physical attributes are a more or less finite number of parameters. The difference is how you interpret it. Let’s say you have bruising — the software will detect the percentage of bruise and then you can use that information to determine, for example, whether or not you should send that strawberry shipment from California to Florida. Or it can tell you how much shelf life is left based on the redness of the fruit.
What happens next, after the software produces its results?
The software produces the results, but then the operator can still decide what to do with those results. It’s like having additional pair of completely unbiased eyes.
Our platform is applicable to wherever the inspections or quality assessments are done visually, from the producer to the processor or the retailer. The assessment can be based on a USDA specification or a customer’s unique specification.
We’re looking at integrations down the road. For example, we’ll be able to integrate the software with sorters and conveyor belt systems in processing facilities. This would make sorting during the quality and grade assessments absolutely seamless.
Why did you choose to build a mobile-first solution?
All you need is a smartphone to use our entire platform. I’ve been in the mobile space for more than a decade, even before mobile was a space. I’m very biased about using mobile as an approach, because by now we can safely make the assumption that, no matter what, everyone has a phone.
This is important to me because we want to make AgShift a global solution. Imagine a cashew farm in Africa. If they can use a smartphone to do the quality assessment right at the source, they’ll hopefully be able to get a much better price because they’ll have a much higher quality product. By boosting quality, we can help create value for every stakeholder in the supply chain.
There are actually two steps to automating the inspection process. The deep learning we’ve been talking about is the second one. The first step is digitizing it to begin with. We call this the end-to-end digital workflow platform. The idea is that from the point of doing the inspection to the point of the retail, the whole thing can be digitized.
Think of an organization that has 1000 commodities, like a retailer for all the things you see in the produce section at the grocery store. All of the USDA specifications for every commodity are built into the platform. The inspectors can use the app to enter the scores, and then the data is sent to the cloud, to the central repository for the organization. Then, whatever next steps need to be taken on the basis of that data can be done in a seamless way.
This can increase operational efficiency. Say an organization has inspections happening in Australia or Mexico or Brazil. They can use our app to send the inspection data to a central location and so they can analyze where problems occur. For example, they could identify suppliers whose products regularly fall short of quality thresholds, or they can uncover inconsistencies in the inspection process. That’s the first step, and this platform is ready to go.
The second step is pushing it even further with deep learning to make the whole inspect process autonomous. There, we are 100% ready with strawberries, and we’ll be announcing other commodities very soon.
What message do you have for any companies considering using this technology? Or for other entrepreneurs interested in applying new technologies in the food space?
My two cents to all the players in the supply chain is that they need to be more open-minded. Let innovation come in, as long as that innovation has a very clear value proposition for the organization.
For Silicon Valley entrepreneurs, I say let’s take all of this innovation we’re talking about 24/7 and apply it to food. If we do that, if even a small percentage of all of these bright minds channel their efforts to food and ag, it’s almost guaranteed that five years from now we’ll be in a position to solve the complex challenges we’re staring at today. Especially for women entrepreneurs, this is the perfect time to get in.
Want to learn more? Watch Jha’s talk at this year’s Fancy Food Show: